Agentic RAG using Llama Index
08.07.2024
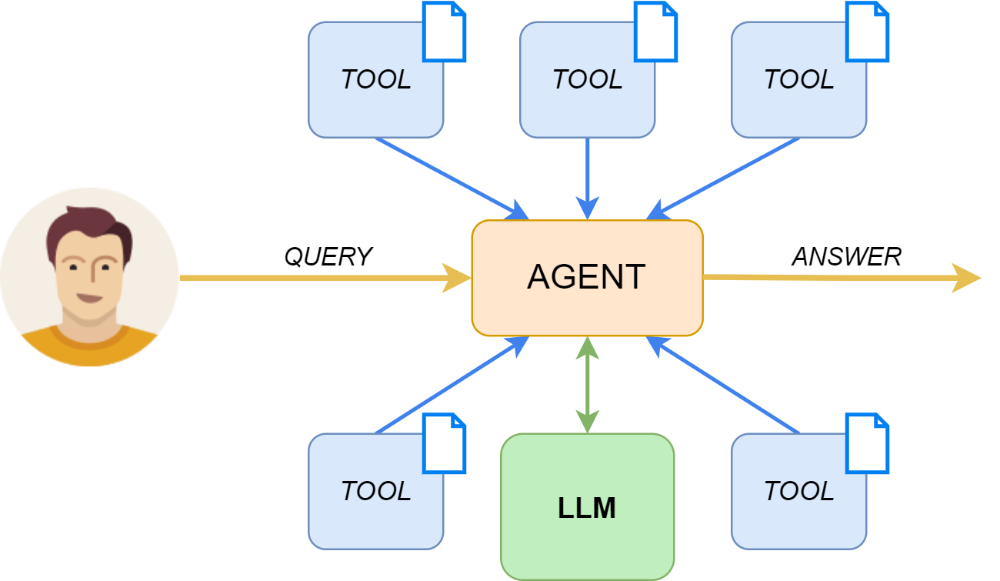
Retrieval-Augmented Generation (RAG) is a technique that combines document retrieval with generative language models to produce precise and contextually accurate responses. Traditional RAG workflows involve a retriever module that searches through a large collection of documents to find relevant ones. These documents are then fed into a generative model, which crafts a coherent answer to the original prompt.
Among the latest advancements in Retrieval-Augmented Generation (RAG), a novel approach called Agentic RAG has emerged. This method surpasses the traditional technique by using an agent equipped with multiple tools, each one dedicated to a specific document, offering a scalable and efficient solution for managing large volumes of documents. When a query is made, the agent evaluates which tools are most suitable to answer it based on relevance. The selected tools retrieve the necessary information which is finally used to generate the response.
To build the Agentic RAG system, these components from Llama Index are used:
- VectorStoreIndex is created for each document, to map its content into a latent vector space and enable efficient retrieval of relevant information;
- ObjectIndex aggregates all the VectorStoreIndex instances and serves as a centralized repository;
- FunctionCallingAgentWorker is created to use the ObjectIndex, this agent is responsible for generating the responses by querying the appropriate tools.
Employing an agent-based approach offers several advantages:
- Modularity: each tool manages a specific set of documents, making the system more flexible and easier to update;
- Flexibility: multiple tools can be engaged for a single query, extracting relevant parts from various documents which are then combined by the agent to provide comprehensive answers.
- Efficient generation: each tool refines individually its retrieved documents, allowing the agent to generate responses combining already polished text segments and avoiding the need for a long single context in one LLM call.
This innovative method not only enhances performance but also allows for more flexible and complex information retrieval and generation. If you want to learn more, have a look at this course from DeepLearning.AI.